What would happen if you had all the time in the world to build a forecast for the next year? Enough time so that not a single thing is left out. Site traffic, conversion rates, hiring, inventory, and every item in between factored into an elaborate prediction model.
Then, 6 months in — BAM! Your forecast is 100% precise. Number by number, line by line; everything is spot on.
You’d likely respond by double-checking every formula, just to make sure there aren’t any errors. Because this never happens in the world of accounting.
Not only is this scenario highly unlikely, but attempting to achieve this level of numerical enlightenment could actually be bad for business.
You read that right.
It might seem counterintuitive, but this level of precision is not the point of financial modeling, and it can even harm your forecasting capabilities. Instead, we should aim for accuracy.
To do that, we need to understand the difference between precision and accuracy.
What is precision vs. accuracy?
Granted these two words are similar in nature and definition. That said, there is a difference, particularly for controllers and CFOs.
Here’s an example from real life: I was once tasked with building a forecast for a medical device company to help pitch for a round of funding. A major goal of the forecast was to predict the impact of switching manufacturing from in-house to outsourced production.
I eagerly got to work.
In order to precisely account for every possible number of units sold, and the related cost and profitability, I created a model that tabulated every conceivable Cost of Goods Sold (COGS) in 1000 unit increments.
And I did it up to 300,000 units sold.
The result was a massive, 36 MB Excel workbook. It was so large and complex because the various levels of possible production filtered back into the financial forecast and multiple other sheets in the file.
The model was precise down to:
- The exact number of units sold
- Each cost to produce those units (accounting for discounts at each 1000 unit tier)
- The tabulation in the overall forecast
The problem with precision
There was one glaring problem with my incredibly precise forecast. The problem wasn’t the number of metrics. Or the complex breakdown of costs.
The problem was that no one else understood how to update or even use it.
The model was stuffed into the supporting documentation of the overall investment presentation. And when it came time for potential investors to see how the numbers on the slides were calculated—the Excel spreadsheets wouldn’t work for them.
Even if the investors could get them running, it would be nearly impossible for me to explain succinctly how they worked.
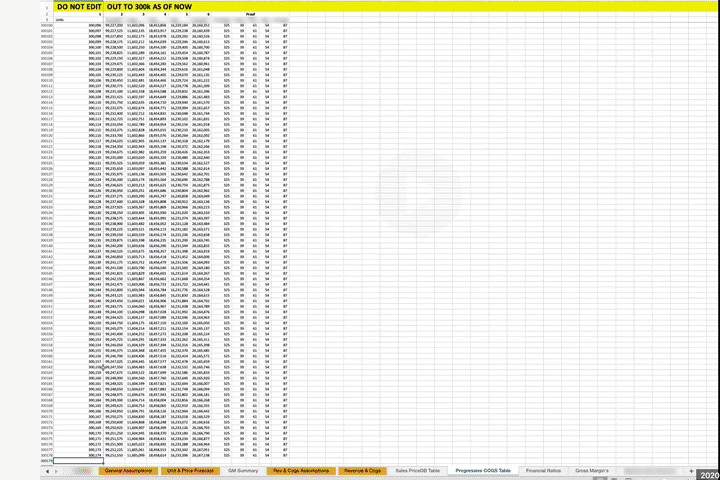
Here's a glimpse at a portion of my overly-precise financial model. That's over 300,000 rows in one sheet!
The solution? A simpler, yet still accurate model.
Long-term accuracy is far more effective than short-term precision
An accurate financial model is one that:
- Allows for everyone (who needs to) to understand how the numbers work, not just what the numbers are
- Is easily updated, so it remains useful over a set period of time, instead of quickly becoming unusable or inaccurate
- Allows for easy decision-making thanks to clarity and ease-of-use — not necessarily 100% precision
Ideally, a financial model should be simple enough for anyone on the executive team to update.
Business leaders — not just finance leaders — should be able to quickly change the quantity of goods and cost of goods assumptions without needing a detailed explanation of how things work.
This is the big problem with precision. The more precise your model, the more complex your calculations, and so fewer people (if any) will understand how you came to your conclusions.
That barrier to entry in understanding the model results in an inaccurate forecast over time.
In my own example, no one knew how to operate the workbook and even if they did, it was unreliable due to the size of the file. If I’d made the model simpler, those potential investors would have been able to see the business potential for themselves instead of having to trust my slides in the pitch deck.
Here are a few more ways accuracy wins over precision:
- Makes the model more understandable and usable by those who are using it
- A model regularly updated is more reliable than a “precise” one-time forecast
- With a better understanding of the model, better business decisions can be made
Complexity might be fun, but simplicity wins in the long run
A simpler model is often a more accurate model over time. And that should be the goal — and the reason for existing — for any financial model.
Key takeaway: When building a financial forecast or financial model, you may be tempted to try to get everything perfect. This requires a lot of variables, assumptions and guesswork to get it right. When this happens, you spend a ton of time, create a lot of complexity, and ultimately hurt your ability to forecast. So instead of trying to be so precise, you should try to be accurate. Accept that there will be a margin of error in your forecast. And make the model easy to share and update, even for non-finance experts.
How to create a more accurate forecast
There are lots of reasons for creating a financial model. You may want to pitch investors, prepare for a sale of your business, improve cash flow, or just improve overall profitability. Whatever the situation, use an accurate forecast. And be careful not to get too precise.
Here’s another quick example of how accuracy wins over precision:
A company is figuring out how many sales reps to hire over the next 3 to 5 years. To do this, they need to project the commission each sales rep will earn.
Option A: Average the average commission payout percentage and multiply this by sales. For instance, if the company has two commission tiers (one at 10% and another at 20%), we blend the two rates and forecast a 15% commission payout in aggregate.
Option B: A more precise way to forecast commission expense is to calculate how much each rep could make… for every month. This requires building out a large, complex table of hires, rates, and months. Then you pull the totals back into your financial forecast. The result is a bulky model that no one wants to use a few months down the road because it’s too difficult to figure out how it works and have confidence that there aren’t errors.
Option A won’t forecast commission expense to the dollar, but it will probably be pretty close, and you’ll likely actually use and update the model with Option A. If the forecasted commission expense isn’t matching up with reality, then it’s a simple task to change the average commission percentage assumption.
Less precision doesn’t mean less data
And no, sacrificing precision doesn’t mean using fewer metrics or oversimplifying data.
For example, with Jirav you’re able to input any number of variables, track key KPIs, and even add custom spreadsheets to your analysis and forecasts. Reports and financial dashboards are easily updated and make the numbers easy to understand for anyone.
Start a free trial today and see for yourself how accuracy wins over precision for financial modeling.